Estimation and Mapping Above-Ground Mangrove Carbon Stock Using Sentinel-2 Data Derived Vegetation Indices in Benoa Bay of Bali Province, Indonesia
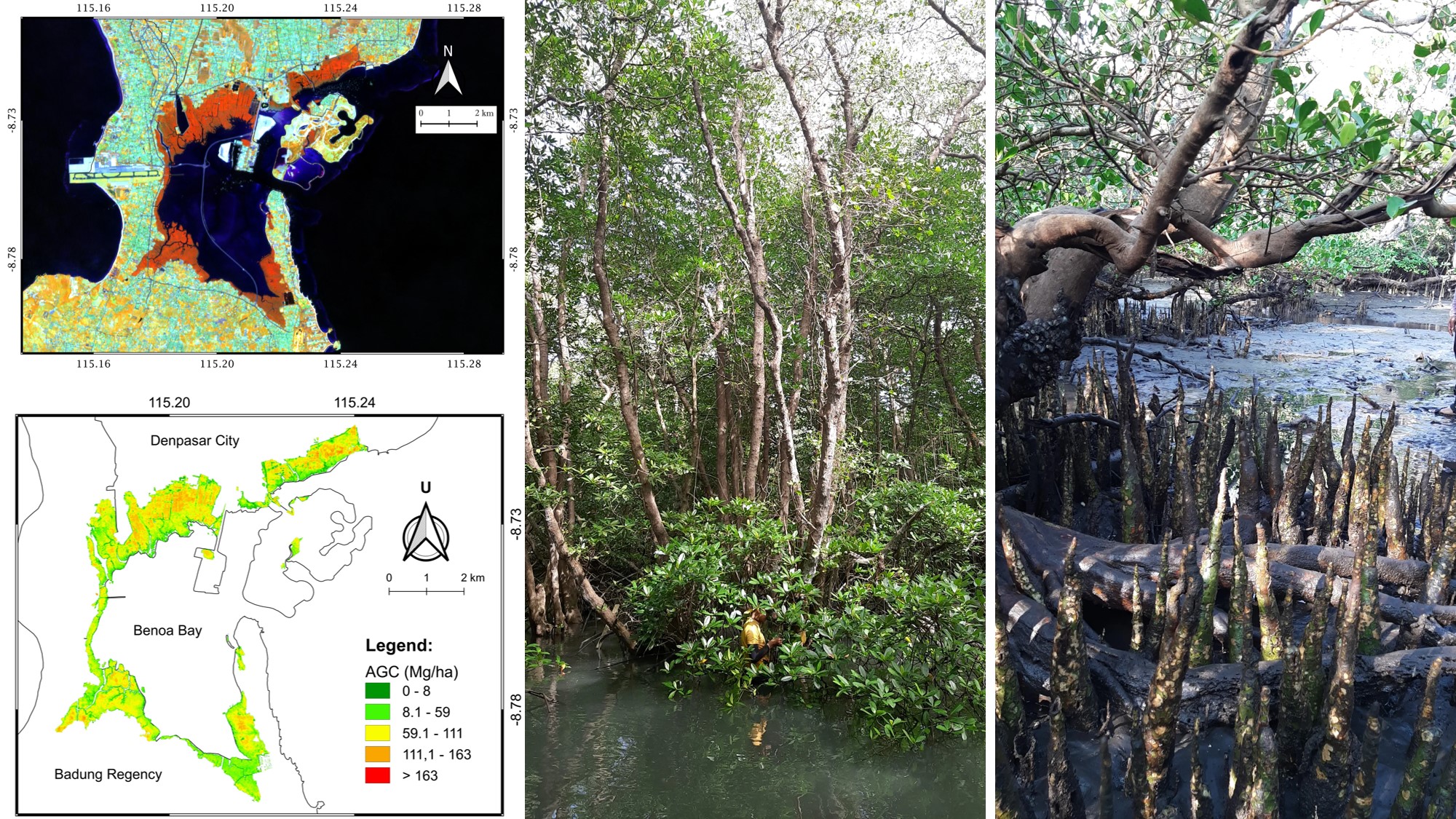
Additional Files
Carbon dioxide (CO2) is one of the greenhouse gases that causes global warming with the highest concentration in the atmosphere. Mangrove forests can absorb CO2 three times higher than terrestrial forests and tropical rainforests. Moreover, mangrove forests can be a source of Indonesian income in the form of a blue economy, therefore an accurate method is needed to investigates mangrove carbon stock. Utilization of remote sensing data with the results of the above-ground carbon (AGC) detection model of mangrove forests based on multispectral imaging and vegetation index, can be a solution to get fast, cheap, and accurate information related to AGC estimation. This study aimed to investigates the best model for estimating the AGC of mangroves using Sentinel-2 imagery in Benoa Bay, Bali Province. The random forest (RF) method was used to classified the difference between mangrove and non-mangrove with the treatment of several parameters. Furthermore, a semi-empirical approach was used to assessed and map the AGC of mangroves. Allometric equations were used to calculated and produced AGC per species. Moreover, the model was built with linear regression equations for one variable x, and multiple regression equations for more than one x variable. Root Mean Square Error (RMSE) was used to assess the validation of the model results. The results of the mangrove forests area detected in the research location around 1134.92 ha, with an Overall Accuracy (OA) of 0.984 and a kappa coefficient of 0.961. This study highlights that the best model was the combination of IRECI and TRVI vegetation indices (RMSE: 11.09 Mg/ha) for a model based on red edge bands. Meanwhile, the best results from the model that does not use the red edge band were the combination of TRVI and DVI vegetation indices (RMSE: 13.63 Mg/ha). The use of red edge and NIR bands is highly recommended in building the AGC model of mangrove forests because they can increase the accuracy value. Thus, the results of this study are highly recommended in estimating the AGC of mangrove forests, because it has been proven to be able to increase the accuracy value of previous studies using optical images.
Abdul-Hamid, H., Mohamad-Ismail, F. N., Mohamed, J., Samdin, Z., Abiri, R., Tuan-Ibrahim, T. M., ... & Naji, H. R. (2022). Allometric equation for aboveground biomass estimation of mixed mature mangrove forest. Forests, 13(2), 1–18. https://doi.org/10.3390/f13020325
Alongi, D. M. (2012). Carbon sequestration in mangrove forests. Carbon Management, 3(3), 313–322. https://doi.org/10.4155/cmt.12.20
Breiman, L. (2001). Random Forests. Machine Learning, 45, 5–32. https://doi.org/ 10.14569/ijacsa.2016.070603
Brown, S. (2002). Measuring carbon in forests: Current status and future challenges. Environmental Pollution, 116(3), 363–372. https://doi.org/10.1016/S0269-7491(01)00212-3
Castillo, J. A. A., Apan, A. A., Maraseni, T. N., & Salmo, S. G. (2017). Estimation and mapping of above-ground biomass of mangrove forests and their replacement land uses in the Philippines using Sentinel imagery. ISPRS Journal of Photogrammetry and Remote Sensing, 134, 70–85. https://doi.org/10.1016/ j.isprsjprs.2017.10.016
Cerón-Souza, I., Rivera-Ocasio, E., Medina, E., Jiménez, J. A., McMillan, W. O., & Bermingham, E. (2010). Hybridization and introgression in new world red mangroves, Rhizophora (Rhizophoraceae). American Journal of Botany, 97(6), 945–957. https://doi.org/10.3732/ajb.0900172
Chai, T., & Draxler, R. R. (2014). Root mean square error (RMSE) or mean absolute error (MAE)? -Arguments against avoiding RMSE in the literature. Geoscientific Model Development, 7(3), 1247–1250. https://doi.org/10.5194/gmd-7-1247-2014
Clevers, J. G. P. W., Jong, S. M. De, Epema, G. F., Addink, E. a, & Box, P. O. (2000). Meris and the Red-Edge Index. 2nd EARSeL Workshop, Enschede.
Curran, P. J., Windham, W. R., & Gholz, H. L. (1995). Exploring the relationship between reflectance red edge and chlorophyll concentration in slash pine leaves. Tree Physiology, 15(3), 203–206. https://doi.org/10.1093/treephys/15.3.203
Dan, T. T., Chen, C. F., Chiang, S. H., & Ogawa, S. (2016). Mapping and Change Analysis in Mangrove Forest By Using Landsat Imagery. ISPRS Annals of Photogrammetry, Remote Sensing and Spatial Information Sciences, III–8(July), 109–116. https://doi.org/10.5194/isprsannals-iii-8-109-2016
Dewanti, L. P. P., Subagiyo, & Wijayanti, D. P. (2020). Analysis of Biomass and Stored Carbon Stock in Mangrove Forest Area, Taman Hutan Raya Ngurah Rai Bali. Indonesian Journal of Fisheries Science and Technology, 16(3), 219–224.
Dong, S., Chen, Z., Gao, B., Guo, H., Sun, D., & Pan, Y. (2020). Stratified even sampling method for accuracy assessment of land use/land cover classification: a case study of Beijing, China. International Journal of Remote Sensing, 41(16), 6427–6443. https://doi.org/10.1080/01431161.2020.1739349
Dube, T., Gara, T. W., Mutanga, O., Sibanda, M., Shoko, C., Murwira, A., ... & Hatendi, C. M. (2018). Estimating forest standing biomass in savanna woodlands as an indicator of forest productivity using the new generation WorldView-2 sensor. Geocarto International, 33(2), 178–188. https://doi.org/10.1080/10106049. 2016.1240717
ESA. (2012). Sentinel-2: ESA’s Optical High-Resolution Mission for GMES Operational Services.
ESA. (2015). Sentinel-2 User Handbook. In ESA Standard Document Date (Issue 1). https://doi.org/10.1021/ie51400a018
Fadaei, H., Suzuki, R., Sakai, T., & Torii, K. (2012). a Proposed New Vegetation Index, the Total Ratio Vegetation Index (Trvi), for Arid and Semi-Arid Regions. The International Archives of the Photogrammetry, Remote Sensing and Spatial Information Sciences, XXXIX-B8 (September), 403–407. https://doi.org/ 10.5194/isprsarchives-xxxix-b8-403-2012
FAO. (2007). The world’s mangroves 1980-2005. In FAO Forestry Paper (Vol. 153).
Foody, G. M., Cutler, M. E., McMorrow, J., Pelz, D., Tangki, H., Boyd, D. S., & Douglas, I. A. N. (2001). Mapping the biomass of Bornean tropical rain forest from remotely sensed data. Global Ecology & Biogeography, 10(4), 379–387. https://doi.org/ 10.1046/j.1466-822X.2001.00248.x
Fourqurean, J. W., Johnson, B., Kauffman, J. B., Kennedy, H., Lovelock, C. E., Megonigal, J. P., Rahman, A., Saintilan, N., & Simard, M. (2019). Coastal Blue Carbon. Habitat Conservation, Ci, 860. http://www.habitat.noaa.gov/coastalbluecarbon.html
Fromard, F., Puig, H., Mougin, E., Marty, G., Betoulle, J. L., & Cadamuro, L. (1998). Structure, above-ground biomass and dynamics of mangrove ecosystems: New data from French Guiana. Oecologia, 115(1–2), 39–53. https://doi.org/10.1007/ s004420050489
Gitelson, A. A., Gritz, Y., & Merzlyak, M. N. (2003). Relationships between leaf chlorophyll content and spectral reflectance and algorithms for non-destructive chlorophyll assessment in higher plant leaves. Journal of Plant Physiology, 160(3), 271–282. https://doi.org/10.1078/0176-1617-00887
Gitelson, A. A., Keydan, G. P., & Merzlyak, M. N. (2006). Three-band model for noninvasive estimation of chlorophyll, carotenoids, and anthocyanin contents in higher plant leaves. Geophysical Research Letters, 33(11), 2–6. https://doi.org/ 10.1029/2006GL026457
Goswami, J., Das, R., Sarma, K. K., & Raju, P. L. N. (2021). Red Edge Position (REP), an Indicator for Crop Stress Detection: Implication on Rice (Oryza sativa L). International Journal of Environment and Climate Change, December, 88–96. https://doi.org/10.9734/ijecc/2021/v11i430396
Hallik, L., Kuusk, A., Lang, M., & Kuusk, J. (2019). Reflectance properties of hemiboreal mixed forest canopies with focus on red edge and near infrared spectral regions. Remote Sensing, 11(14). https://doi.org/10.3390/rs11141717
Han, J., Kamber, M., & Pei, J. (2012). Data Mining Concepts and Techniques (3rd Edition). Elsevier. https://doi.org/10.1016/C2009-0-61819-5
Hastuti, A. W., Suniada, K. I., & Islamy, F. (2017). Carbon Stock Estimation of Mangrove Vegetation Using Remote Sensing in Perancak Estuary, Jembrana District, Bali. International Journal of Remote Sensing and Earth Sciences (IJReSES), 14(2), 137. https://doi.org/10.30536/j.ijreses.2017.v14.a2841
Heumann, B. W. (2011). Satellite remote sensing of mangrove forests: Recent advances and future opportunities. Progress in Physical Geography, 35(1), 87–108. https://doi.org/10.1177/0309133310385371
Hirata, Y., Tabuchi, R., Patanaponpaiboon, P., Poungparn, S., Yoneda, R., & Fujioka, Y. (2014). Estimation of aboveground biomass in mangrove forests using high-resolution satellite data. Journal of Forest Research, 19(1), 34–41. https:// doi.org/10.1007/s10310-013-0402-5
Hong-wei, Z., Huai-liang, C., & Fei-na, Z. (2019). The Modification of Difference Vegetation Index (DVI) in middle and late growing period of winter wheat and its application in soil moisture inversion. E3S Web of Conferences, 131. https://doi.org/10.1051/e3sconf/201913101098
Iksan, M., Al Zarliani, W. O. D., Nare, L., Hafidhawati, S., & Baena, F. (2019). Biomass and Carbon Uptake of Mangrove Forests Pohorua Village, Muna Regency. International Journal of Applied Biology, 3(2), 57–64.
Imran, H. A., Gianelle, D., Rocchini, D., Dalponte, M., Martín, M. P., Sakowska, K., Wohlfahrt, G., & Vescovo, L. (2020). VIS-NIR, red-edge and NIR-shoulder based normalized vegetation indices response to co-varying leaf and Canopy structural traits in heterogeneous grasslands. Remote Sensing, 12(14). https://doi.org/ 10.3390/rs12142254
Indonesian National Standard (SNI). (2011). Pengukuran dan Penghitungan Cadangan Karbon – Pengukuran Lapangan untuk Penaksiran Cadangan Karbon Hutan ( Ground Based Forest Carbon Accounting ).
Jachowski, N. R. A., Quak, M. S. Y., Friess, D. A., Duangnamon, D., Webb, E. L., & Ziegler, A. D. (2013). Mangrove biomass estimation in Southwest Thailand using machine learning. Applied Geography, 45, 311–321. https://doi.org/10.1016/j.apgeog. 2013.09.024
Jordan, C. F. (1969). Derivation of Leaf-Area Index From Quality of Light on Forest Floor. Ecological Society of America, 50(4), 663–666. https://doi.org/205.133.226.104
Kauffman, J. B., & Cole, T. G. (2010). Micronesian mangrove forest structure and tree responses to a severe typhoon. Wetlands, 30(6), 1077–1084. https://doi.org/ 10.1007/s13157-010-0114-y
Kauffman, J. B., & Donato, D. C. (2012). Protocols for the measurement, monitoring and reporting of structure, biomass and carbon stocks in mangrove forests. Working Paper 86. CIFOR, Bogor, Indonesia.
Komiyama, A., Ong, J. E., & Poungparn, S. (2008). Allometry, biomass, and productivity of mangrove forests: A review. Aquatic Botany, 89(2), 128–137. https://doi.org/10.1016/j.aquabot.2007.12.006
Komiyama, A., Poungparn, S., & Kato, S. (2005). Common allometric equations for estimating the tree weight of mangroves. Journal of Tropical Ecology, 21(4), 471–477. https://doi.org/10.1017/S0266467405002476
Kumar, L., & Mutanga, O. (2017). Remote sensing of above-ground biomass. Remote Sensing, 9(9), 1–8. https://doi.org/10.3390/rs9090935
Li, X., Yeh, A. G. O., Wang, S., Liu, K., Liu, X., Qian, J., & Chen, X. (2007). Regression and analytical models for estimating mangrove wetland biomass in South China using Radarsat images. International Journal of Remote Sensing, 28(24), 5567–5582. https://doi.org/10.1080/01431160701227638
Mahasani, I. G. A. I., Osawa, T., Adnyana, I. W. S., Suardana, A. A. M. A. P., & Chonnaniyah. (2021). Carbon stock estimation and mapping of mangrove forest using ALOS-2 PALSAR-2 in Benoa Bay Bali, Indonesia. IOP Conference Series: Earth and Environmental Science, 2(1), 429–443. https://doi.org/10.1088/1755-1315/ 944/1/012044
Mahasani, I. I., Osawa, T., & Sandi Adnyana, I. W. (2021). Estimation and Mapping of Above Ground Biomass of Mangrove Forest Using Alos-2 Palsar-2 in Benoa Bay, Bali, Indonesia. ECOTROPHIC : Jurnal Ilmu Lingkungan, 15(1), 75. https://doi.org/10.24843/ejes.2021.v15.i01.p07
Murdiyarso, D., Purbopuspito, J., Kauffman, J. B., Warren, M. W., Sasmito, S. D., Donato, D. C., ... & Kurnianto, S. (2015). The potential of Indonesian mangrove forests for global climate change mitigation. Nature Climate Change, 5(12), 1089–1092. https://doi.org/10.1038/ nclimate2734
Nguy-Robertson, A. L., Peng, Y., Gitelson, A. A., Arkebauer, T. J., Pimstein, A., Herrmann, I., ... & Bonfil, D. J. (2014). Estimating green LAI in four crops: Potential of determining optimal spectral bands for a universal algorithm. Agricultural and Forest Meteorology, 192–193, 140–148. https://doi.org/10.1016/j.agrformet. 2014.03.004
Nguyen, H. H., Tran, L. T. N., Le, A. T., Nghia, N. H., Duong, L. V. K., Nguyen, H. T. T., ... & Premnath, C. F. S. (2020). Monitoring changes in coastal mangrove extents using multi-temporal satellite data in selected communes, Hai Phong City, Vietnam. Forest and Society, 4(1), 256–270. https://doi.org/10.24259/fs.v4i1.8486
Oshiro, T. M., Perez, P. S., & Baranauskas, J. A. (2012). How many trees in a random forest? Conference: 8th International Conference on Machine Learning and Data Mining in Pattern Recognition, MLDM 2012, Lecture Notes in Computer Science, 7376 LNAI(July 2012), 154–168. https://doi.org/10.1007/978-3-642-31537-4_13
Patil, V., Singh, A., Naik, N., & Unnikrishnan, S. (2015). Estimation of Mangrove Carbon Stocks by Applying Remote Sensing and GIS Techniques. Wetlands, 35(4), 695–707. https://doi.org/10.1007/s13157-015-0660-4
Pearson, T., Walker, S., & Brown, S. (2005). Sourcebook for Land use , Land-use change and forestry Projects. Retrieved from https://www.winrock.org/wp-content/uploads/2016/03/Winrock-BioCarbon_Fund_Sourcebook-compressed.pdf
Pham, L. T. H., & Brabyn, L. (2017). Monitoring mangrove biomass change in Vietnam using SPOT images and an object-based approach combined with machine learning algorithms. ISPRS Journal of Photogrammetry and Remote Sensing, 128, 86–97. https://doi.org/10.1016/j.isprsjprs.2017.03.013
Pham, T. D., Yoshino, K., Le, N. N., & Bui, D. T. (2018). Estimating aboveground biomass of a mangrove plantation on the Northern coast of Vietnam using machine learning techniques with an integration of ALOS-2 PALSAR-2 and Sentinel-2A data. International Journal of Remote Sensing, 39(22), 7761–7788. https://doi.org/10.1080/01431161.2018.1471544
Proisy, C., Couteron, P., & Fromard, F. (2007). Predicting and mapping mangrove biomass from canopy grain analysis using Fourier-based textural ordination of IKONOS images. Remote Sensing of Environment, 109(3), 379–392. https://doi.org/10.1016/j.rse.2007.01.009
Pu, R., & Landry, S. (2012). A comparative analysis of high spatial resolution IKONOS and WorldView-2 imagery for mapping urban tree species. Remote Sensing of Environment, 124, 516–533. https://doi.org/10.1016/j.rse.2012.06.011
Purnamasari, E., Kamal, M., & Wicaksono, P. (2021). Comparison of vegetation indices for estimating above-ground mangrove carbon stocks using PlanetScope image. Regional Studies in Marine Science, 44. https://doi.org/10.1016/j.rsma.2021. 101730
Ramdani, F., Rahman, S., & Giri, C. (2018). Principal polar spectral indices for mapping mangroves forest in South East Asia: study case Indonesia. International Journal of Digital Earth, 12(10), 1103–1117. https://doi.org/10.1080/17538947. 2018.1454516
Regier, P., Duggan, M., Myers-Pigg, A., & Ward, N. (2023). Effects of random forest modeling decisions on biogeochemical time series predictions. Limnology and Oceanography: Methods, 21(1), 40–52. https://doi.org/10.1002/lom3.10523
Richards, D. R., & Friess, D. A. (2016). Rates and drivers of mangrove deforestation in Southeast Asia, 2000-2012. Proceedings of the National Academy of Sciences of the United States of America, 113(2), 344–349. https://doi.org/10.1073/pnas. 1510272113
Rodriguez-Galiano, V. F., Ghimire, B., Rogan, J., Chica-Olmo, M., & Rigol-Sanchez, J. P. (2012). An assessment of the effectiveness of a random forest classifier for land-cover classification. ISPRS Journal of Photogrammetry and Remote Sensing, 67(1), 93–104. https://doi.org/10.1016/j.isprsjprs.2011.11.002
Rouse, J. W., Haas, R. H., Schell, J. A., & Deering, D. W. (1973). Monitoring Vegetation Systems In The Great Plains With Erts. In Remote Sensing Center. https://doi.org/10.1021/jf60203a024
Sadeghi, M., Jones, S. B., & Philpot, W. D. (2015). A linear physically-based model for remote sensing of soil moisture using short wave infrared bands. Remote Sensing of Environment, 164, 66–76. https://doi.org/10.1016/j.rse.2015.04.007
Savage, N. H., Agnew, P., Davis, L. S., Ordóñez, C., Thorpe, R., Johnson, C. E., O’Connor, F. M., & Dalvi, M. (2013). Air quality modelling using the Met Office Unified Model (AQUM OS24-26): Model description and initial evaluation. Geoscientific Model Development, 6(2), 353–372. https://doi.org/10.5194/gmd-6-353-2013
Sibanda, M., Mutanga, O., & Rouget, M. (2015). Examining the potential of Sentinel-2 MSI spectral resolution in quantifying above ground biomass across different fertilizer treatments. ISPRS Journal of Photogrammetry and Remote Sensing, 110, 55–65. https://doi.org/10.1016/j.isprsjprs.2015.10.005
Sidik, F., Fernanda Adame, M., & Lovelock, C. E. (2019). Carbon sequestration and fluxes of restored mangroves in abandoned aquaculture ponds. Journal of the Indian Ocean Region, 15(2), 177–192. https://doi.org/10.1080/19480881.2019. 1605659
Suardana, A. M. A. P., Anggraini, N., Aziz, K., Nandika, M. R., Ulfa, A., Wijaya, A. D., ... & Dewanti, R. (2022). Biomass Estimation Model and Carbon Dioxide Sequestration for Mangrove Forest Using Sentinel-2 in Benoa Bay, Bali. International Journal of Remote Sensing and Earth Sciences, 19(1), 91–100.
Suwa, R., Rollon, R., Sharma, S., Yoshikai, M., Albano, G. M. G., Ono, K., ... & Nadaoka, K. (2021). Mangrove biomass estimation using canopy height and wood density in the South East and East Asian regions. Estuarine, Coastal and Shelf Science, 248(May), 106937. https://doi.org/10.1016/j.ecss. 2020.106937
Tucker, C. J. (1980). A spectral method for determining the percentage of green herbage material in clipped samples. Remote Sensing of Environment, 9(2), 175–181. https://doi.org/10.1016/0034-4257(80)90007-3
Wang, B., Jia, K., Liang, S., Xie, X., Wei, X., Zhao, X., Yao, Y., & Zhang, X. (2018). Assessment of Sentinel-2 MSI spectral band reflectances for estimating fractional vegetation cover. Remote Sensing, 10(12), 1–20. https://doi.org/ 10.3390/rs10121927
Wicaksono, P., Danoedoro, P., Hartono, H., Nehren, U., & Ribbe, L. (2011). Preliminary work of mangrove ecosystem carbon stock mapping in small island using remote sensing: above and below ground carbon stock mapping on medium resolution satellite image. Remote Sensing for Agriculture, Ecosystems, and Hydrology XIII, 8174(October 2011), 81741B. https://doi.org/10.1117/12.897926
Wicaksono, P., Danoedoro, P., Hartono, & Nehren, U. (2016). Mangrove biomass carbon stock mapping of the Karimunjawa Islands using multispectral remote sensing. International Journal of Remote Sensing, 37(1), 26–52. https://doi.org/ 10.1080/01431161.2015.1117679
World Agroforestry Center. (2022). Wood density. Accessed from http://db.worldagroforestry.org/wd
Zhu, Y., Liu, K., Liu, L., Myint, S. W., Wang, S., Liu, H., & He, Z. (2017). Exploring the potential of world view-2 red-edge band-based vegetation indices for estimation of mangrove leaf area index with machine learning algorithms. Remote Sensing, 9(10). https://doi.org/10.3390/rs9101060
Copyright (c) 2023 Forest and Society

This work is licensed under a Creative Commons Attribution 4.0 International License.
This is an open access journal which means that all contents is freely available without charge to the user or his/her institution. Users are allowed to read, download, copy, distribute, print, search, or link to the full texts of the articles in this journal without asking prior permission from the publisher or the author. This is in accordance with the BOAI definition of open access.
Submission of an article implies that the work described has not been published previously (except in the form of an abstract or as part of a published lecture or academic thesis), that it is not under consideration for publication elsewhere, that its publication is approved by all authors and tacitly or explicitly by the responsible authorities where the work was carried out, and that, if accepted, will not be published elsewhere in the same form, in English or in any other language, without the written consent of the Publisher. An article based on a section from a completed graduate dissertation may be published in Forest and Society, but only if this is allowed by author's(s') university rules. The Editors reserve the right to edit or otherwise alter all contributions, but authors will receive proofs for approval before publication.
Forest and Society operates a CC-BY 4.0 © license for journal papers. Copyright remains with the author, but Forest and Society is licensed to publish the paper, and the author agrees to make the article available with the CC-BY 4.0 license. Reproduction as another journal article in whole or in part would be plagiarism. Forest and Society reserves all rights except those granted in this copyright notice