Correlation of Climate Variability and Burned Area in Borneo using Clustering Methods
Versions
- 2020-09-13 (2)
- 2020-07-29 (1)
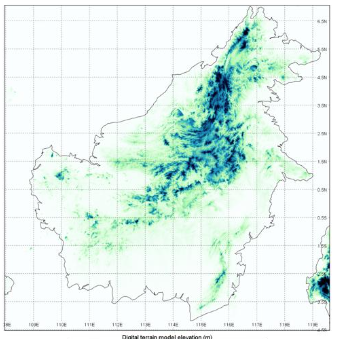
The island of Borneo has faced seasonal forest fires for decades. This phenomenon is worsening during dry seasons, especially when droughts are concurrent with the El Niño-Southern Oscillation (ENSO) phenomenon. Climate is therefore one of the drivers of the fire phenomenon. This paper studies the relationship between climate variables, namely temperature, precipitation, relative humidity, and wind speed, and the occurrence of forest fire using two clustering methods, K-means and Fuzzy C-means (FCM) clustering methods. Borneo is clustered into four areas based on burned area data obtained from Global Fire Emission Data (GFED). It is also clustered according to the combinations of climate variables. Both methods reach the highest correlation between the climate variable and the burned area clusters in September. The K-means method gives a correlation of -0.54 while the FCM gives -0.55. In August until October, relative humidity provides the dominant correlation affecting burned area, even though an additional precipitation or wind variable slightly increases the correlation in the FCM method. In November, temperature largely contributed to the burned area by a positive correlation of 0.31 in K-means and 0.33 in FCM. The evaluation performance of the methods is conducted by an internal validation called the Silhouette index. Both methods have positive index values ranging from 0.39 to 0.69 and the maximum value is influenced by the wind cluster. This indicates that the clustering methods applied in this paper can identify one or a combination of climate variables into dense and well-separated clusters.
Aflahah, E., Hidayati, R., Hidayat, R., & Alfahmi, F. (2018). Pendugaan hotspot sebagai indikator kebakaran hutan di Kalimantan berdasarkan faktor iklim Hotspot asummption as a forest fire indicator in Kalimantan based on climate factor. Journal of Natural Resources and Environmental Management, 9(2), 405–418. doi: https://doi.org/https://doi.org/10.29244/jpsl.9.2.405-418
Aryadi, M., Satriadi, T., & Syam’ani, S. (2018). KECENDERUNGAN KEBAKARAN HUTAN DAN LAHAN DAN ALTERNATIF PENGENDALIAN BERBASIS KEMITRAAN DI PT. INHUTANI II KOTABARU Trend of Forest and Land Fire and Control Alternative Based on Partnership at PT. Inhutani II Kotabaru. Jurnal Hutan Tropis, 5(3), 222. doi: https://doi.org/10.20527/jht.v5i3.4789
Barber, C. V., & Schweithelm, J. (2000). Trial by fire: Forest fires and forestry policy in Indonesia’s era of crisis and reform. Washington D.C, USA, World Resources Institute (WRI), Forest Frontiers Initiative. In collaboration with WWF-Indonesia and Telapak Indonesia Foundation.
Bezdek, J. C. (1981). Pattern Recognition with Fuzzy Objective Function Algorithms. doi: https://doi.org/10.1007/978-1-4757-0450-1
Bezdek, J. C., Ehrlich, R., & Full, W. (1984). FCM: The fuzzy c-means clustering algorithm. Computers and Geosciences, 10(2–3), 191–203. doi: https://doi.org/10.1016/0098-3004(84)90020-7
Dunn, J. C. (1973). A fuzzy relative of the ISODATA process and its use in detecting compact well-separated clusters. Journal of Cybernetics, 3(3), 32–57. doi: https://doi.org/10.1080/01969727308546046
Fadholi, A. (2013). Studi Dampak El Nino Dan Indian Ocean Dipole (Iod) Terhadap Curah Hujan Di Pangkalpinang. Jurnal Ilmu Lingkungan, 12(2), 43. doi:https://doi.org/10.14710/jil.11.1.43-50
Ganesan, P., Sathish, B. S., & Sajiv, G. (2016). A comparative approach of identification and segmentation of forest fire region in high resolution satellite images. IEEE WCTFTR 2016 - Proceedings of 2016 World Conference on Futuristic Trends in Research and Innovation for Social Welfare, 3–8. doi: https://doi.org/10.1109/STARTUP.2016.7583959
Ghosh, S., & Kumar, S. (2013). Comparative Analysis of K-Means and Fuzzy C-Means Algorithms. International Journal of Advanced Computer Science and Applications, 4(4), 35–39. doi: https://doi.org/10.14569/ijacsa.2013.040406
Giglio, L., Randerson, J. T., & Van Der Werf, G. R. (2013). Analysis of daily, monthly, and annual burned area using the fourth-generation global fire emissions database (GFED4). Journal of Geophysical Research: Biogeosciences, 118(1), 317–328. doi: https://doi.org/10.1002/jgrg.20042
Jafarzadeh, A. A., Mahdavi, A., & Jafarzadeh, H. (2017). Evaluation of forest fire risk using the Apriori algorithm and fuzzy c-means clustering. Journal of Forest Science, 63(8), 370–380. doi: https://doi.org/10.17221/7/2017-JFS
Kementerian Lingkungan Hidup dan Kehutanan Republik Indonesia. (2018). Berita Negara. In Peraturan Menteri Lingkungan Hidup dan Kehutanan Republik Indonesia Nomor P.9/MENLHK/SETJEN/KUM.1/3/2018 Tentang Kriteria Teknis Status Kesiagaan dan Darurat Kebakaran Hutan dan Lahan. Retrieved from http://ditjenpp.kemenkumham.go.id/arsip/bn/2018/bn375-2018.pdf
Kita, K., Fujiwara, M., & Kawakami, S. (2000). Total ozone increase associated with forest fires over the Indonesian region and its relation to the El Nino-Southern oscillation. Atmospheric Environment, 34(17), 2681–2690. doi: https://doi.org/10.1016/S1352-2310(99)00522-1
Konca-Kędzierska, K., & Pianko-Kluczyńska, K. (2019). The influence of relative humidity on fires in forests of Central Poland. Forest Research Papers, 79(3), 269–279. doi: https://doi.org/10.2478/frp-2018-0027
Latifah, A. L., Shabrina, A., Wahyuni, I. N., & Sadikin, R. (2019). Evaluation of Random Forest model for forest fire prediction based on climatology over Borneo. 2019 International Conference on Computer, Control, Informatics and Its Applications (IC3INA), 4–8. doi: https://doi.org/10.1109/IC3INA48034.2019.8949588
Lengyel, A., & Botta-Dukát, Z. (2019). Silhouette width using generalized mean—A flexible method for assessing clustering efficiency. Ecology and Evolution, 9(23), 13231–13243. doi: https://doi.org/10.1002/ece3.5774
Maimon, O., & Rokach, L. (2010). Data Mining and Knowledge Discovery Handbook. doi: https://doi.org/10.1007/978-0-387-09823-4
Mareta, L., Hidayat, R., Hidayati, R., & Latifah, A. L. (2019). Pengaruh Faktor Alami dan Antropogenik Terhadap Luas Kebakaran Hutan dan Lahan di Kalimantan. Jurnal Tanah Dan Iklim, 43(2), 143–151. doi: https://doi.org/10.2017/jti.v43i2.10456
Margono, B. A., Turubanova, S., Zhuravleva, I., Potapov, P., Tyukavina, A., Baccini, A., … Hansen, M. C. (2012). Mapping and monitoring deforestation and forest degradation in Sumatra (Indonesia) using Landsat time series data sets from 1990 to 2010. Environmental Research Letters, 7(3). doi: https://doi.org/10.1088/1748-9326/7/3/034010
Natingga, D. (2017). Data Science Algorithms in a Week. Retrieved from https://www.packtpub.com/big-data-and-business-intelligence/data-science-algorithms-week
Pramesti, D. F., Lahan, Tanzil Furqon, M., & Dewi, C. (2017). Implementasi Metode K-Medoids Clustering Untuk Pengelompokan Data. Jurnal Pengembangan Teknologi Informasi Dan Ilmu Komputer, 1(9), 723–732. doi: https://doi.org/10.1109/EUMC.2008.4751704
Prasad, K. S. N., & Ramakrishna, S. (2008). An autonomous forest fire detection system based on spatial data mining and fuzzy logic. IJCSNS International Journal of Computer Science and Network Security, 8(12), 49–55. Retrieved from http://paper.ijcsns.org/07_book/200812/20081208.pdf
Pratamasari, A., Permatasari, N. K. F., Pramudiyasari, T., Manessa, M. D. M., & Supriatna, S. (2020). Spatial Distribution Patterns Analysis of Hotspot in Central Kalimantan using FIMRS MODIS Data. Jurnal Geografi Lingkungan Tropik, 4(1), 24–34. doi: https://doi.org/10.7454/jglitrop.v4i1.74
Putra, Erianto I, Vetrita, Y., & Graham, L. L. B. (2016). Degraded peatlands, Ground Water Level and severe peat fire occurrences. International Peat Congress, (August).
Putra, Erianto Indra, & Hayasaka, H. (2011). The effect of the precipitation pattern of the dry season on peat fire occurrence in the Mega Rice Project area, Central Kalimantan, Indonesia. Tropics, 19(4), 145–156. doi: https://doi.org/10.3759/tropics.19.145
Putra, Erianto Indra, Hayasaka, H., Takahashi, H., & Usup, A. (2008). Recent Peat Fire Activity in the Mega Rice Project Area, Central Kalimantan, Indonesia. Journal of Disaster Research, 3(5), 334–34. doi: https://doi.org/10.20965/jdr.2008.p0334
Rao, V. S., & Vidyavathi, S. (2010). Comparative Investigations and Performance Analysis of Fcm and Mfpcm Algorithms on Iris Data. Indian Journal of Computer Science and Engineering, 1(2), 145–151. Retrieved from https://www.ijcse.com/docs/IJCSE10-01-02-12.pdf
Rousseeuw, P. J. (1987). Silhouettes: A graphical aid to the interpretation and validation of cluster analysis. Journal of Computational and Applied Mathematics, 20(C), 53–65. doi: https://doi.org/10.1016/0377-0427(87)90125-7
Sari, N. M., Rachmita, N., & Manessa, M. D. M. (2020). Hotspot Distribution Analysis In East Kalimantan Province 2017-2019 to Support Forest and Land Fires Mitigation. Indonesian Journal of Environmental Management and Sustainability, 4(1), 28–33. doi: https://doi.org/10.26554/ijems.2020.4.1.28-33
Shidik, G. F., & Mustofa, K. (2014). Predicting Size of Forest Fire. In Lecture Notes in Computer Science (pp. 316–327). doi: https://doi.org/10.1007/978-3-642-55032-4_31
Shoolihah, A.-M., Furqon, M. T., & Widodo, A. W. (2017). Implementasi Metode Improved K-Means untuk Mengelompokkan Titik Panas Bumi. Jurnal Pengembangan Teknologi Informasi Dan Ilmu Komputer (J-PTIIK) Universitas Brawijaya, 1(11), 1270–1276. Retrieved from http://j-ptiik.ub.ac.id/index.php/j-ptiik/article/view/453
Siegert, F., & Hoffmann, A. A. (2000). The 1998 forest fires in East Kalimantan (Indonesia): A quantitative evaluation using high resolution, multitemporal ERS-2 SAR images and NOOA-AVHRR hotspot data. Remote Sensing of Environment, 72(1), 64–77. doi: https://doi.org/10.1016/S0034-4257(99)00092-9
Sukamto, S., Id, I. D., & Angraini, T. R. (2018). Penentuan Daerah Rawan Titik Api di Provinsi Riau Menggunakan Clustering Algoritma K-Means. JUITA : Jurnal Informatika, 6(2), 137. doi: https://doi.org/10.30595/juita.v6i2.3172
Suyanto. (2018). Machine Learning: Tingkat Dasar dan Lanjut. Retrieved from http://biobses.com/product/machine-learning-tingkat-dasar-dan-lanjut/
Syaufina, L., & Sukmana, A. (2008). Tinjauan PenyebabUtama Kebakaran Hutan di Daerah Tangkapan Air Danau Toba. In ITTO PROJECT PD 394/06 REV.1 (F) Centre of Forest and Nature Conservation Research and Development (CFNCRD).
Tacconi, L., Moore, P. F., & Kaimowitz, D. (2007). Fires in tropical forests - What is really the problem? Lessons from Indonesia. Mitigation and Adaptation Strategies for Global Change, 12(1), 55–66. doi: https://doi.org/10.1007/s11027-006-9040-y
Usup, A., Hashimoto, Y., Takahashi, H., & Hayasaka, H. (2004). Combustion and thermal characteristics of peat fire in tropical peatland in Central Kalimantan, Indonesia. Tropics, 14(1), 1–19. doi: https://doi.org/10.3759/tropics.14.1
Wang, Z., Liu, P., & Cui, T. (2017). Research on forest flame recognition algorithm based on image feature. International Archives of the Photogrammetry, Remote Sensing and Spatial Information Sciences - ISPRS Archives, 42(2W7), 925–928. doi: https://doi.org/10.5194/isprs-archives-XLII-2-W7-925-2017
Wu, Z., He, H. S., Fang, L., Liang, Y., & Parsons, R. A. (2018). Wind speed and relative humidity influence spatial patterns of burn severity in boreal forests of northeastern China. Annals of Forest Science, 75(3). doi: https://doi.org/10.1007/s13595-018-0749-z
Xu, T. S., Chiang, H. D., Liu, G. Y., & Tan, C. W. (2017). Hierarchical K-means Method for Clustering Large-Scale Advanced Metering Infrastructure Data. IEEE Transactions on Power Delivery, 32(2), 609–616. doi: https://doi.org/10.1109/TPWRD.2015.2479941
Yong, Y., Chongxun, Z., & Pan, L. (2004). A novel fuzzy c-means clustering algorithm for image thresholding. Measurement Science Review, 4(1), 11–19. Retrieved from http://www.measurement.sk/2004/S1/Yong.pdf
Copyright (c) 2020 Forest and Society

This work is licensed under a Creative Commons Attribution 4.0 International License.
This is an open access journal which means that all contents is freely available without charge to the user or his/her institution. Users are allowed to read, download, copy, distribute, print, search, or link to the full texts of the articles in this journal without asking prior permission from the publisher or the author. This is in accordance with the BOAI definition of open access.
Submission of an article implies that the work described has not been published previously (except in the form of an abstract or as part of a published lecture or academic thesis), that it is not under consideration for publication elsewhere, that its publication is approved by all authors and tacitly or explicitly by the responsible authorities where the work was carried out, and that, if accepted, will not be published elsewhere in the same form, in English or in any other language, without the written consent of the Publisher. An article based on a section from a completed graduate dissertation may be published in Forest and Society, but only if this is allowed by author's(s') university rules. The Editors reserve the right to edit or otherwise alter all contributions, but authors will receive proofs for approval before publication.
Forest and Society operates a CC-BY 4.0 © license for journal papers. Copyright remains with the author, but Forest and Society is licensed to publish the paper, and the author agrees to make the article available with the CC-BY 4.0 license. Reproduction as another journal article in whole or in part would be plagiarism. Forest and Society reserves all rights except those granted in this copyright notice